Interpreting Chinese Statistics: Extracting Expenditure-side Quarter-on-quarter Growth Contributions
china, consumption, export, investment, modelling
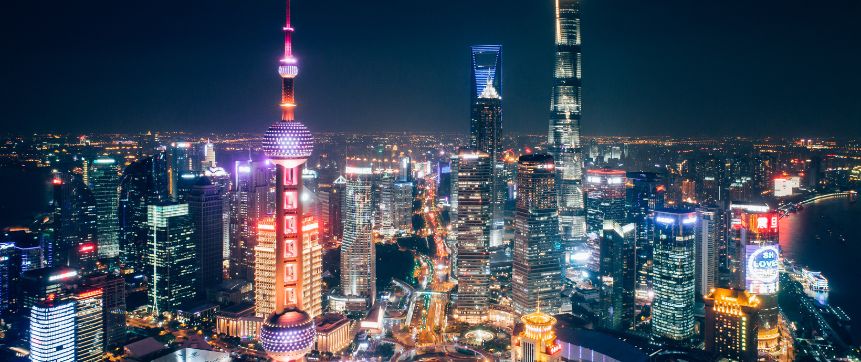
Photo: IanZ – Getty Images
Abstract
Components for GDP on the expenditure side of the national accounts – expenditure on consumption, investment (including inventories) and exports less imports – can provide an important read on the composition of demand. For China, these components are available in contributions to year-ended GDP growth, which provides insight into trends but makes it difficult to interpret how the economy is operating quarter to quarter. This article discusses a method for deriving contributions to quarter-on-quarter GDP growth using official data that allows for a better understanding of expenditure side drivers of quarter to quarter. The decomposition shows that strong growth in the March quarter of 2024 was driven by a large increase in net exports, but growth in the June quarter was mainly supported by investment, which likely reflected a large contribution from the change in inventories. This suggests that Chinese domestic demand remained sluggish in the first half of the year, despite the strong outcome for GDP growth in the March quarter.
Introduction
The National Bureau of Statistics (NBS) – Chinas national statistics agency – publishes more detail on the production side of the national accounts in their estimate of quarterly GDP growth than the expenditure side, and does not publish expenditure contributions to quarter-on-quarter (qoq) GDP growth or qoq growth of the expenditure components of GDP. Information on the composition of demand within each quarter – the split between expenditure on consumption, investment and exports less imports – provides a useful read on how the Chinese economy is operating, and has implications for our view of the outlook. For example, the qoq growth rates of these expenditure components can help us understand, in a timely manner, whether GDP growth is being driven by domestic or external demand.
Growth in different components can also have different implications for Australia. For example, strong investment growth could imply strong steel and thus iron ore demand, while higher consumption growth could imply more demand for tourism or luxury goods. Although the NBS provides expenditure components in annual national accounts data, and as contributions to year-ended growth (published every quarter), these do not provide a direct read on qoq growth.
In this article, we provide a method for deriving contributions to qoq GDP growth using official NBS data. This decomposition allows us to better understand expenditure side drivers of quarter to quarter, and also to better detect turning points in Chinese domestic demand and risks to growth.
Overview
To attribute GDP growth from quarter to quarter to the underlying contributions from consumption, investment (including inventories) and (net) exports, we use a simple method to calculate contributions to seasonally adjusted (SA) qoq GDP growth from non-seasonally adjusted (NSA) contributions to year-ended GDP growth. This method can be thought of as a direct conversion from underlying NBS data, with minimal error introduced. Our calculated expenditure side contributions to SA qoq GDP growth will not completely match the headline NBS series of SA qoq GDP growth, due to our contributions being derived using our own seasonal adjustment process rather than NBS seasonal adjustments, but the differences are generally small.
After we have obtained the contributions from total consumption and total investment to quarterly GDP growth, we use other data to estimate a further breakdown of these components into household and government consumption, and investment and inventories. We also derive monthly trade indices from year-ended data as a cross-check of our net exports contribution to GDP.
Constructing expenditure side contributions to quarter-on-quarter growth
To construct expenditure contributions from consumption, investment and net exports to SA qoq growth series, we use contributions to year-ended growth data (Graph 1; as published by the NBS every quarter) and annual nominal shares of expenditure components (Graph 2). Consumption here is a broad measure that includes household and government consumption, and investment is gross capital formation that includes changes in inventories. This construction occurs in four steps:
- Calculate year-ended growth rates for consumption and investment for every quarter from published contributions to year-ended growth data and nominal shares of expenditure components.
- Use a seasonal adjustment trick to convert year-ended growth of consumption and investment to SA qoq growth rates of these components.
- Use SA qoq growth rates together with the nominal shares of consumption and investment to calculate contributions to quarterly GDP growth for these two components.
- Calculate, as a residual, net exports.
Code for the process described is available on request.
Graph 1
Graph 2
Step 1: Calculate year-ended growth rates
To calculate year-ended growth rates for each expenditure component for each quarter from their quarterly contributions to year-ended growth, we use the formula for contributions to real GDP growth for consumption and investment (component i) and solve for real year-ended growth (Equation 1):
Ideally, we would use the nominal share of each component from the base quarter of the year-ended growth calculation (i.e. the share four quarters ago), but quarterly shares are not published by the NBS. However, the shares change very slowly from year to year (Graph 2), so we simply use the annual nominal share from the year prior. To provide a guide to the size of error this could introduce, we identify the largest change in consumption and investment shares from year to year since 2000 – a change in the investment share of 4.7 percentage points from 2008 to 2009. We then assess how sensitive our calculated year-ended growth series for investment and consumption are to varying the weights by that amount, and find that the resulting change in annual shares, shown by the bands in Graph 3, is reasonably small.
Graph 3
We cannot directly calculate the contribution from net exports using Equation 1 because we do not have individual contributions from imports and exports (see Appendix A for why this matters). Therefore, we assume the net exports contribution is the residual once the contributions of consumption and investment to growth have been accounted for.
Step 2: Convert year-ended growth rates to SA quarter-on-quarter growth rates
Once we have year-ended growth rates for consumption and investment, we make use of a simple property of seasonal adjustment:
After seasonal adjustment using X-13ARIMA-SEATS, a qoq or month-on-month (mom) growth profile constructed from year-ended growth data is invariant to the choice of base year values used to construct the profile (to a close approximation).
The method only requires us to choose arbitrary values for a base year, grow them forward using year-ended growth, and then seasonally adjust that series. For more discussion on this trick, see Appendix B.
We choose 2014 as our base year with each quarter in 2014 set to 100 and seasonally adjust using X-13ARIMA-SEATS. Using the resulting index, we then calculate qoq growth rates (Graph 4; Graph 5).
Graph 4
Graph 5
Steps 3 and 4: Construct quarterly contributions and calculate net exports
Finally, we use the annual nominal expenditure shares of GDP to calculate contributions to quarterly growth for consumption and investment, which is Equation 1 in reverse (but using shares in the current year). Then, net exports is calculated as a residual (Graph 6):
Because the net exports contribution is calculated as a residual, it captures any error in the conversion of investment and consumption to year-ended growth and any misalignment in seasonal adjustment between investment, consumption and our SA GDP series (aggregating SA subcomponents of a series does not in general give the same result as seasonally adjusting the aggregate series). We minimise this misalignment by using the same seasonal adjustment settings for investment and consumption and using our own headline SA qoq GDP growth series, , rather than using NBS SA qoq growth (see Appendix C for more detail). The difference in seasonal adjustment of qoq GDP growth between the NBS and the RBA is shown in grey in Graph 6.
Graph 6
Breaking down consumption and investment further
The quarterly contributions from consumption and investment estimated using the approach described above are for total consumption and total investment (i.e. gross capital formation, GCF). Total consumption includes both household and government consumption, and GCF includes both gross fixed capital formation (GFCF) and change in inventories. We further decompose growth in total consumption and GCF into these components. However, to obtain these decompositions, we have to draw signal from partial data that introduces much greater uncertainty into this decomposition compared with the one discussed above.
Consumption
We can estimate the contribution from household consumption to total consumption growth using survey data on household consumption from Chinas quarterly Household Income, Expenditure and Living Conditions Survey (Household Survey) as a proxy for consumption in the national accounts. Government consumption can then be estimated as a residual from total consumption. Household consumption from the Household Survey is released in nominal, per capita, NSA adjusted terms, so we first need to convert it into real, total, SA terms using the following steps:
- multiply by population, interpolated from the NBS annual series of total population
- deflate using the NBS Consumer Price Index (CPI)
- seasonally adjust, in this case using the same seasonal adjustment parameters used in the broad expenditure side components discussed above.
Using annual, nominal GDP shares of household consumption and total consumption, we calculate the share of household consumption in total consumption using Equation 1 (but using the household share of total consumption in the current year), with government consumption as the residual (Graph 7). This breakdown is an estimate with more significant sources of error than the estimate of total consumption growth. Real household consumption growth calculated from the Household Survey is subject to considerable error, and is different from the national accounts measure due to sampling and methodological differences. For example, household consumption in the national accounts not only includes goods and services bought by households directly, but also includes goods and services obtained in other ways, such as goods and services produced and consumed by households themselves.
Graph 7
Investment (gross capital formation)
We can estimate the components of gross capital formation (GCF) – gross fixed capital formation (GFCF) and change in inventories – by using nominal monthly fixed asset investment (FAI) data as a proxy for growth GFCF, and then estimating the contribution from the change in inventories as a residual from the total investment qoq growth series derived above. Nominal FAI data are scaled to account for conceptual differences between nominal FAI and nominal GFCF, and converted into real terms using a weighted average of producer price indices (PPIs) as a deflator. Then, using Equation 1 (but using GFCFs share of total investment in the current year), we calculate the contribution to GCF from GFCF, with the contribution from the change in inventories as the residual of total investment (Graph 8). This decomposition shows that the large positive contribution to growth from gross capital formation in the June quarter of 2024 was mostly from change in inventories. Due to differences between FAI and GFCF, these estimates are subject to considerable uncertainty. However, these estimates are still useful as a summary of our best estimate of the contributions of GFCF and the change in inventories to quarterly growth in China. Further details on these estimates are given in Appendix D.
Graph 8
Diving deeper into net exports
We compare our net exports estimates against Chinas General Administration of Customs (China Customs) data on merchandise trade volumes and prices (CNY basis) by converting their year-ended growth data to a level index using seasonal adjustment as described in Appendix B (Graph 9). This allows us to better understand month-to-month movements and offers a useful qualitative cross-check on our above estimate of the contribution of net exports to qoq growth (as merchandise trade data from China Customs do not include services trade). The resulting indices suggest that merchandise export volumes increased significantly in the first quarter of 2024, and increased by more than the merchandise values series suggest, as export prices declined at the same time. A large increase in export volumes aligns with the strong contribution from net exports in our GDP growth decomposition over the same timeframe.
Graph 9
Conclusion
We have shown in this article that we can derive expenditure side contributions to qoq growth from the data provided by the NBS. This decomposition highlights recent key drivers of growth. Net exports and household consumption were the key drivers of the strong outcome in the first quarter of this year as external demand ramped up quickly and there was strong spending by households on domestic tourism during the Lunar New Year holiday (Graph 10). In the second quarter, these drivers waned and qoq growth declined. It was largely investment that kept GDP growth positive – but this was likely supported by a large contribution from change in inventories that does not necessarily imply higher steel demand.
Finally, we note that these estimates are subject to changing seasonal factors, so could change over time as more data arrives. These revisions could be larger than usual for some time as seasonal patterns reassert themselves or change in the post-pandemic period.
Graph 10
Appendix A: Contribution from net exports
The contribution from net exports would be calculated as follows:
Having the contribution to net exports only makes it impossible to separately identify real year-ended growth in exports and imports.
Appendix B: Seasonal adjustment trick
Extracting quarter-on-quarter growth from year-ended growth data
Consider Chinas headline year-ended GDP growth for quarter t applied to different arbitrary profiles in the base year:
where (the first four quarters) is set to some sensible arbitrary value.
The quarterly index profiles grown forward from different arbitrary base years are completely different (Graph B.1, second panel). However, after seasonal adjustment, qoq growth is indistinguishable (fourth panel). In other words, when growing forward arbitrary values in a base year using year-ended growth rates, the resulting NSA profile in levels is meaningless, but the SA qoq growth rate series is not (this can also be presented as a re-based levels index).
Graph B.1
Harris and Yilmaz (2008) show that the SA qoq growth rates from the derived series are exactly the same as the SA qoq growth rates from the true series when using seasonal adjustment techniques that have linearly separable seasonal factors (e.g. those with constant seasonal factors).
Using more complicated algorithms where seasonal factors can change over time and are therefore not linearly separable, such as those implemented in X-13ARIMA-SEATS, could introduce some error, but Harris and Yilmaz (2008) show that this is minimal using UK retail sales data where the actual series is known.
Below we provide two examples where the underlying series is known (i.e. using Australian and Chinese data) and we perform simulations involving a synthetic seasonal break on Chinese GDP data. These examples and simulations show that the seasonal adjustment property holds up well when using X-13ARIMA-SEATS.
Examples of two known series
To demonstrate that our method uncovers reasonably accurate qoq or mom growth rates, we apply it to two data series for which we know the base year (Graph B.2; Graph B.3). In each case, we replace the actual data in the first year with 100 for each quarter or month and follow our method to recover qoq or mom growth rates by growing forward with year-ended growth rates and then seasonally adjusting. The Australian Bureau of Statistics (ABS) series that we seasonally adjust will not be the same as the official ABS SA series, as the seasonal adjustment method is not the same.
Graph B.2
Graph B.3
Generalising further
Is it possible to generalise, particularly in a situation where the seasonal factors change? We run some basic simulations to indicate where the method might fail when using X-13ARIMA-SEATS.
The NBS publish real GDP as an NSA levels index so we can use the true base year and a very different arbitrary base year to see what happens when using X-13ARIMA-SEATS when there is a large seasonal break. We perform simulations where, in each iteration, we change the base year and add a synthetic seasonal break. This is not an exhaustive test of this method, but indicates when care is needed in applying the method.
Base year
In each iteration, we scale all quarters in the original base year by a random number drawn from a uniform distribution where = U(-a,a) (Table B.1). We choose a=0.2 and a=0.4 in separate simulations. These produce a range of large changes in the base year.
Quarter | Adjusted base year |
---|---|
Q1 | |
Q2 | |
Q3 | |
Q4 |
Synthetic seasonal break
We insert the same synthetic seasonal break in each iteration in both series by multiplying the index in quarters 1, 2 and 3 from March 2020 onwards by a factor and quarter 4 by (Table B.2). For each iteration, is chosen from a uniform distribution of U(–b,b). This creates a synthetic seasonal break in just one quarter. We choose b=0 (no synthetic break), b=0.1 and b=0.2 in separate simulations. These distributions create a range of large seasonal breaks.
Original with seasonal break | Adjusted with seasonal break |
---|---|
Simulations
We run six different simulations, each with n =100 (Table B.3).
Simulation | a (base year) | b (seasonal break) |
---|---|---|
1 | 0.2 | 0 |
2 | 0.2 | 0.1 |
3 | 0.2 | 0.2 |
4 | 0.4 | 0 |
5 | 0.4 | 0.1 |
6 | 0.4 | 0.2 |
We find errors to be reasonably small, except around the seasonal break when b =0.2 (Graph B.4).
Graph B.4
Picking out just one draw from a=0.4, b=0.2, we can see that despite a very different base year and the large seasonal break that causes significant changes in qoq growth, the adjusted base year series and original growth series remain reasonably close (Table B.4; Graph B.5).
Original base year |
Adjusted base year |
Seasonal break |
---|---|---|
100 | 84 | 0.90 |
115 | 97 | 1.08 |
129 | 118 | 0.83 |
143 | 166 | 1.20 |
Graph B.5
When using this method, we do not observe the true underlying base year, so we never know how much of an impact the error in our assumed base year might have. However, we can detect large seasonal breaks by looking at a time series of seasonal factors (in a seasonal/irregular plot) over time. We do not observe changes in seasonal factors in the series used in this article that are large enough to cause concern.
In some iterations, the automatic outlier detection in X-13ARIMA-SEATS selects different outliers for series with different base years, which can cause material differences. Manually imposing outliers when there are strong priors around what they should be would minimise this source of error, but without knowing the underlying series it may be hard to say what the outliers should be. Ultimately, an outlier should be discarded if there is no reasonable explanation for the outlier.
Appendix C: Comparing RBA and NBS seasonal adjustment settings
We choose to seasonally adjust headline GDP ourselves to align the method of seasonal adjustment between total GDP growth with its components. The difference between our constructed SA qoq GDP growth series and that published by the NBS is not large, which is unsurprising, as X-13ARIMA-SEATS also forms the basis for NBS seasonal adjustment (Graph C.1; NBS 2023). There are some bigger differences in quarter 2 of 2021 and quarter 2 of 2023, but statistical agencies commonly made interventions through the COVID-19 pandemic period, whereas we do not make such interventions here.
Graph C.1
The settings used in the R seasonal package for each of our NSA indices of GDP, consumption and investment is given below (Sax and Eddelbuettel 2018). Since net exports is calculated as a residual from these three variables, we align the outliers and arima model for the three series to preserve addivity as best as possible when using direct seasonal adjustment, as described by the ONS (2007). We align outliers by taking the union of outliers between investment and consumption models when outliers are auto selected, and align ARIMA models by taking the highest order model from investment and consumption:
library(seasonal)
# GDP.level.constructed.nsa, C.level.constructed.nsa and
# GCF.level.constructed.nsa are r time series objects.
sa_model.GDP <- seas( x = GDP.level.constructed.nsa, x11 = "", regression.variables = c("const", "ao2020.1", "ao2020.2", "ao2022.2"), arima.model = "(1 0 1)(0 1 0)", regression.aictest = NULL, outlier = NULL, transform.function = "log"
)
sa_model.C <- seas( x = C.level.constructed.nsa, x11 = "", regression.variables = c("const", "ao2020.1", "ao2020.2", "ao2022.2"), arima.model = "(1 0 1)(0 1 0)", regression.aictest = NULL, outlier = NULL, transform.function = "log" )
sa_model.GCF <- seas( x = GCF.level.constructed.nsa, x11 = "", regression.variables = c("const", "ao2020.1", "ao2020.2", "ao2022.2"), arima.model = "(1 0 1)(0 1 0)", regression.aictest = NULL, outlier = NULL, transform.function = "log"
)
Appendix D: Investment (GCF) estimates
We decompose GCF growth in four steps:
- Estimate a growth scaling factor that we use to estimate quarterly growth in nominal GFCF from quarterly growth in nominal fixed asset investment (FAI), which is an alternative measure of investment published monthly by the NBS.
- Apply the scaling factor to nominal quarterly FAI growth.
- Convert from nominal estimate to real estimate.
- Calculate contributions to GCF.
Step 1: Estimate a growth scaling factor
FAI and GFCF are two nominal measures of investment published by the NBS. FAI is published monthly, while GFCF is published only annually. The NBS state that they use FAI to estimate GFCF in the national accounts, so it is reasonable to assume they are closely related. But unadjusted, the two measures diverge significantly from one another. There are several reasons for this gap. Conceptual differences between FAI and GFCF – mainly the inclusion of land sales and purchases of existing assets in FAI – mean that FAI should be larger than GFCF. However, these differences do not appear large enough to fully explain the gap between the two measures. The NBS has noted in the past that local authorities sometimes inflate FAI data, which could explain some of the residual error.
We estimate the scaling factor between annual growth in the two measures to be 0.72, which is the geometric average of the ratio between annual GFCF and annual FAI prior to 2017. Prior to 2017, the ratio between the two measures was quite stable, but since 2017 the ratio has been more volatile, as annual growth in nominal GFCF in some years has been much higher than growth in nominal FAI. We assume the ratio will return to be around this long-term average in the future.
Step 2: Apply the scaling factor
We estimate nominal quarterly growth in GCF by multiplying quarterly nominal FAI by the scaling factor calculated above.
Step 3: Convert from nominal to real
We deflate the nominal estimate using a weighted composite of producer price indices. We estimate a weighted average of PPIs that provides a close approximation of the published deflator up to 2019, and then use the same weights to extend the series forward.
Step 4: Calculate contributions to gross capital formation
We multiply our estimate of GFCF growth by GFCFs nominal share of GCF in the current year to estimate its contribution to quarterly growth in GCF. Subtracting this estimated contribution from quarterly growth in GCF yields a residual that we take to be the contribution from the inventories plus any error. Given the scaling factor does not fully adjust for differences between FAI and GFCF, and GFCFs quarterly share of GCF is not equal to its annual share (though the size of the error from this assumption does not look large in other countries), these estimates are subject to considerable uncertainty. However, these estimates are still preferable to the next best alternative, which is to take signal from FAI data directly.
References
ABS (Australian Bureau of Statistics) (2020), Method Changes during the COVID-19 Period, June, available at <https://www.abs.gov.au/articles/methods-changes-during-covid-19-period>.
Harris D and Yilmaz F (2008), Retrieving Seasonally Adjusted Quarterly Growth Rates from Annual Growth Rates that are Reported Quarterly, European Journal of Operational Research, 188(3), pp 846–853.
NBS (National Bureau of Statistics) (2023), 什么是季节调整?[What is Seasonal Adjustment?], available at <https://www.stats.gov.cn/zs/tjws/tjbk/202301/t20230101_1912931.html>.
ONS (Office for National Statistics) (2007), Guide to Seasonal Adjustment with X12-ARIMA, March, available at <https://ec.europa.eu/eurostat/cache/metadata/Annexes/lci_esqrs_uk_an_4.pdf>.
Sax C and Eddelbuettel D (2018), Seasonal Adjustment by X-13ARIMA-SEATS in R, Journal of Statistical Software, 87(11), pp 1–17.
Source: Reserve Bank of Australia [2021]