Both humans and AI hallucinate — but not in the same way
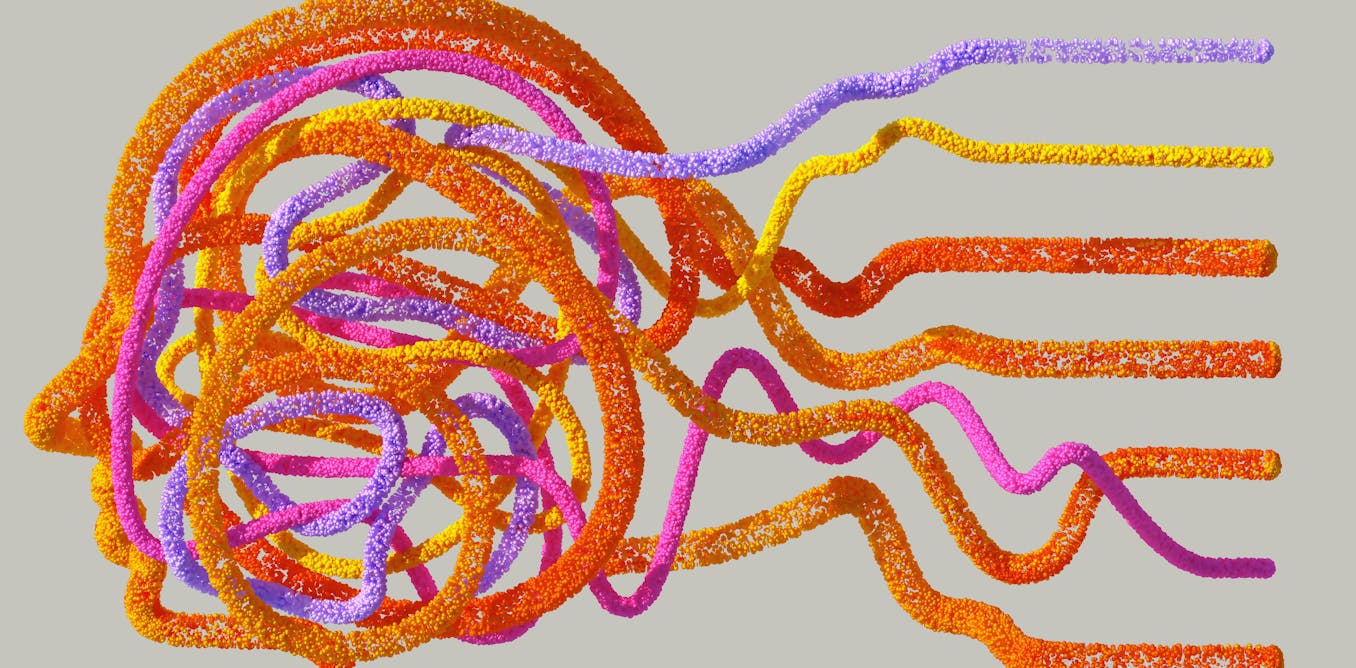
The launch of ever-capable large language models (LLMs) such as GPT-3.5 has sparked much interest over the past six months. However, trust in these models has waned as users have discovered they can make mistakes – and that, just like us, they aren’t perfect.
An LLM that outputs incorrect information is said to be “hallucinating”, and there is now a growing research effort towards minimising this effect. But as we grapple with this task, it’s worth reflecting on our own capacity for bias and hallucination – and how this impacts the accuracy of the LLMs we create.
By understanding the link between AI’s hallucinatory potential and our own, we can begin to create smarter AI systems that will ultimately help reduce human error.
How people hallucinate
It’s no secret people make up information. Sometimes we do this intentionally, and sometimes unintentionally. The latter is a result of cognitive biases, or “heuristics”: mental shortcuts we develop through past experiences.
These shortcuts are often born out of necessity. At any given moment, we can only process a limited amount of the information flooding our senses, and only remember a fraction of all the information we’ve ever been exposed to.
As such, our brains must use learnt associations to fill in the gaps and quickly respond to whatever question or quandary sits before us. In other words, our brains guess what the correct answer might be based on limited knowledge. This is called a “confabulation” and is an example of a human bias.
Our biases can result in poor judgement. Take the automation bias, which is our tendency to favour information generated by automated systems (such as ChatGPT) over information from non-automated sources. This bias can lead us to miss errors and even act upon false information.
Another relevant heuristic is the halo effect, in which our initial impression of something affects our subsequent interactions with it. And the fluency bias, which describes how we favour information presented in an easy-to-read manner.
The bottom line is human thinking is often coloured by its own cognitive biases and distortions, and these “hallucinatory” tendencies largely occur outside of our awareness.
How AI hallucinates
In an LLM context, hallucinating is different. An LLM isn’t trying to conserve limited mental resources to efficiently make sense of the world. “Hallucinating” in this context just describes a failed attempt to predict a suitable response to an input.
Nevertheless, there is still some similarity between how humans and LLMs hallucinate, since LLMs also do this to “fill in the gaps”.
LLMs generate a response by predicting which word is most likely to appear next in a sequence, based on what has come before, and on associations the system has learned through training.
Like humans, LLMs try to predict the most likely response. Unlike humans, they do this without understanding what they’re saying. This is how they can end up outputting nonsense.
As to why LLMs hallucinate, there are a range of factors. A major one is being trained on data that are flawed or insufficient. Other factors include how the system is programmed to learn from these data, and how this programming is reinforced through further training under humans.
Doing better together
So, if both humans and LLMs are susceptible to hallucinating (albeit for different reasons), which is easier to fix?
Fixing the training data and processes underpinning LLMs might seem easier than fixing ourselves. But this fails to consider the human factors that influence AI systems (and is an example of yet another human bias known as a fundamental attribution error).
The reality is our failings and the failings of our technologies are inextricably intertwined, so fixing one will help fix the other. Here are some ways we can do this.
-
Responsible data management. Biases in AI often stem from biased or limited training data. Ways to address this include ensuring training data are diverse and representative, building bias-aware algorithms, and deploying techniques such as data balancing to remove skewed or discriminatory patterns.
-
Transparency and explainable AI. Despite the above actions, however, biases in AI can remain and can be difficult to detect. By studying how biases can enter a system and propagate within it, we can better explain the presence of bias in outputs. This is the basis of “explainable AI”, which is aimed at making AI systems’ decision-making processes more transparent.
-
Putting the public’s interests front and centre. Recognising, managing and learning from biases in an AI requires human accountability and having human values integrated into AI systems. Achieving this means ensuring stakeholders are representative of people from diverse backgrounds, cultures and perspectives.
By working together in this way, it’s possible for us to build smarter AI systems that can help keep all our hallucinations in check.
For instance, AI is being used within healthcare to analyse human decisions. These machine learning systems detect inconsistencies in human data and provide prompts that bring them to the clinician’s attention. As such, diagnostic decisions can be improved while maintaining human accountability.
In a social media context, AI is being used to help train human moderators when trying to identify abuse, such as through the Troll Patrol project aimed at tackling online violence against women.
In another example, combining AI and satellite imagery can help researchers analyse differences in nighttime lighting across regions, and use this as a proxy for the relative poverty of an area (wherein more lighting is correlated with less poverty).
Importantly, while we do the essential work of improving the accuracy of LLMs, we shouldn’t ignore how their current fallibility holds up a mirror to our own.