Can Wage-setting Mechanisms Affect Labour Market Reallocation and Productivity?
business, labour market, productivity, wages
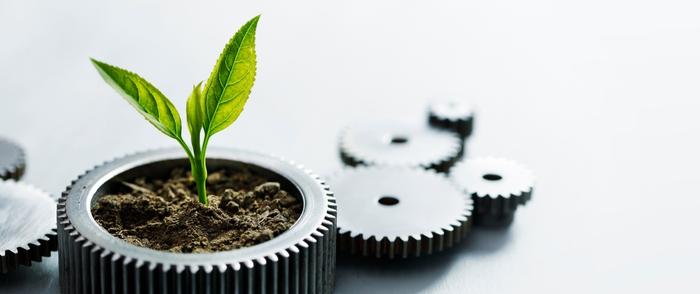
Photo: Ivan Bajic – Getty Images
Abstract
Productivity growth has slowed in Australia and overseas in recent decades, with negative implications for wages and incomes. In Australia, at least part of this slowdown reflects the fact that more productive firms have grown and attracted workers more slowly than in the past. This article considers whether the increased use of industry-wide wage agreements could help to explain this slowdown. It finds that in sectors with greater use of industry-wide agreements, the relationship between firm-level wages and productivity tends to be weaker. This weaker relationship between productivity and wages seems to feed through to firm growth, with more productive firms seemingly less likely to attract staff and grow. While many factors can affect the choice of wage-setting mechanism, these results suggest that aggregate productivity growth and living standards could be stronger when firms are incentivised and able to compete for workers.
Introduction
Productivity measures how much the economy can produce for a given set of inputs (such as labour, capital and land). Over the long term, productivity growth is the key driver of living standards, as it allows economies to produce and consume more for the same amount of inputs – that is, working smarter rather than harder.
Productivity growth can come from two sources:
- individual firms becoming more productive by making better use of their inputs
- more efficient firms growing and attracting labour (and other inputs) at the expense of less efficient firms, often referred to as productivity-enhancing reallocation.
Over recent decades, the pace at which workers tend to move from less to more productive firms in an industry has slowed. This has contributed to slower national productivity growth, and therefore slower growth in national wages and incomes (Andrews and Hansell 2021). It has also meant lower wages for individual workers, as more productive and efficient firms tend to pay higher wages (Card et al 2018). Given the very real implications for both individuals and the economy, it is important to understand why productive firms appear to be growing and attracting workers more slowly in recent decades.
How do more productive firms tend to grow? In some cases they will hire people who are not currently employed. But in many cases they will have to attract, or poach, staff away from other firms by offering them more attractive employment, particularly higher wages (Bilal et al 2022). As such, institutional or regulatory factors that affect the wages that firms offer could also influence the amount of reallocation that occurs.
This could, for example, occur through changes in wage-setting mechanisms, which determine at what level workers and firms bargain and set wages. In recent decades, Australia has had three main wage-setting mechanisms:
- individual arrangements – set between a worker and a firm
- enterprise bargaining agreements (EBA) – set across all workers in a firm
- industry awards (IA) – set across all workers in a role/industry.
For the first two mechanisms, worker wages for the same job can differ across firms. For industry awards, all workers doing the same role will receive the same wage.
In theory, shifts in the prevalence of these wage-setting mechanisms over time could affect the rate of labour reallocation. For example, overseas research has shown that the relationship between firm productivity and wages is weaker where centralised wage-setting mechanisms are used (Guertzgen 2009; OECD 2019; Garnero, Rycx and Terraz 2020). If firms become more likely to offer the same centralised IA wage, there could be less poaching of staff from low to high productivity firms. This might occur if the higher costs incurred in entering non-IA agreements started to outweigh the benefits they provided in terms of flexibility and the ability to attract workers and grow.
That said, it is also possible for increased use of centralised wage-setting mechanisms to cause more labour to flow towards more productive firms. For example, if IA wages, which act as a minimum wage, were too high for low productivity firms to operate profitably, this could cause low productivity firms to shed workers, who might then move to more productive firms (Dustmann et al 2022).
This article examines whether the relationship between productivity and employment growth, and between productivity and wages, differ when different wage-setting mechanisms are used. Such research could potentially shed light on the causes of the slowdown in aggregate productivity growth, and provide more general insights into how wage-setting mechanisms interact with firm- and worker-level outcomes.
Changes in wage-setting mechanisms over time
For wage-setting mechanisms to have contributed to slower reallocation and productivity growth, the prevalence of these mechanisms likely needs to have changed. To consider this, Graph 1 shows the share of workers using IA over time for a number of sectors. While the use of IA declined over the mid-2000s, from around 2010 their use increased. The increase was fairly broadly based across most industries, but experience did differ across industries. For example, the share of workers on IA was broadly flat in the professional services sector, but increased substantially in retail and in accommodation and hospitality. We can exploit these differing outcomes across sectors by looking at whether reallocation slowed more where IA use increased by more.
Graph 1
A look at the link between productivity and growth, and wage-setting mechanisms
To consider the relationships between employment growth and productivity, and between wages and productivity, I used de-identified firm- and worker-level data from the ABS Business Longitudinal Analysis Data Environment (BLADE). This database contains employment and tax data for almost all Australian firms; however, I chose to focus on the non-financial market sector, and so finance, education, public administration and health sector data were excluded from this analysis.
For firms, I constructed measures of employment, wages and labour productivity using taxation data (for more details, see Appendix A). I also drew on the ABS Employee Earning and Hours (EEH) survey microdata, which contains information on workers wages, as well as the wage-setting mechanism under which they are set. I used these to construct worker-, firm- and industry-level measures of the share of workers using different wage-setting mechanisms.
Labour flows to productive firms more slowly when industry award usage goes up
As noted above, the relationship between firm employment growth and productivity could in theory be either stronger or weaker where IA use is more prevalent. This is likely to depend on many different factors, including the level of the IA wage, the share of low and high productivity firms in the economy and the competitiveness of the labour market.
To consider which of these assertions is correct, I regressed firm-level () employment growth on firm productivity (), with productivity expressed as the (log) deviation from the industry average. As such, I compared outcomes for high and low productivity firms in the same industry, and abstracted away from the fact that, for example, mining firms may tend to be more productive than retailers.
More precisely, I ran the following regression:
captures the share of workers in the industry (m) (I used ANZSIC divisions, e.g. mining, retail trade) on IA. I removed the division-level average to focus on changes within industries, rather than differences across industries. This allowed me to abstract from structural differences across industries. For example, IA are heavily used in the retail trade division, which tends to have structurally high labour turnover. I sought to abstract from these structural differences across sectors, which could reflect various other factors, so as to focus on changes that occur alongside changes in IA use. I used either the ABSs published shares or shares constructed from the EEH microdata, as this allowed me to capture different sample periods.
The regression contained a number of additional controls to try to capture other factors that might affect firm employment growth, including firm size and age, sales growth over the previous year, and dummies for each industry in each year to account for industry performance or other structural changes in the industry. I also included an interaction between productivity and state-level unemployment, to account for the fact that the relationship between growth and productivity could be stronger or weaker when the labour market is tight. As such, the regression focuses on structural changes in IA usage, rather than changes driven by labour market strength.
The coefficient of interest is . As discussed above, we generally expect more productive firms to grow more quickly, meaning . But if the relationship between employment growth and productivity is weaker in industries with a higher share of workers on IA, then .
Table 1 shows the results. As expected, more productive firms tend to grow more quickly, as evidenced by the positive coefficient on productivity . However, when more workers are on IA, the relationship between employment growth and productivity tends to weaken, with workers flowing towards more productive firms more slowly . While the weakening is (statistically) insignificant using the published shares over the full sample (column 1), it is significant if 2008 is removed from the sample (column 2). This brings the sample period more in line with the IA shares constructed from the microdata (column 3), where the relationship is again significant. This suggests that the 2008–2010 period may be unusual, potentially reflecting the disruptions of the global financial crisis, or the unusually sharp fall in IA shares in many industries over this period.
Published share – demeaned (1) |
Published share – demeaned (2008 data removed) (2) |
Constructed shares – demeaned (3) |
|
---|---|---|---|
Productivity | 0.032*** | 0.031*** | 0.031*** |
(t-stat) | (14.67) | (14.74) | (15.31) |
Productivity × Award share | −0.026 | −0.050** | −0.047** |
(t-stat) | (−1.41) | (−2.58) | (−2.62) |
Observations | 755,094 | 588,491 | 597,211 |
R-squared | 0.065 | 0.062 | 0.068 |
(a) All regressions include controls for firm demographics (size, age and past sales growth), industry*year FE, and state-level unemployment*productivity (cyclicality of reallocation). *, * and *** show significance at the 10, 5 and 1 per cent level, respectively. Errors clustered at division level (1-digit ANZSIC). Column 1 includes 2008, 2010, 2014 and 2018. Column 2 includes 2010, 2014 and 2018. Column 3 includes 2012, 2014, 2018. Top and bottom percentile of productivity distribution trimmed. Source: RBA |
The coefficients are difficult to interpret directly. As such, a simple scenario based on these results can be derived to illustrate their economic importance, using the implied average employment growth rates for high and low productivity firms and considering how the gap between them would differ for industries with differing IA use. For an industry with average IA use, annual employment growth for high productivity firms is 6.4 percentage points higher than for low productivity firms. Assuming the IA share rose by 5 percentage points, around the average increase from 2010–2018, the gap in employment growth between high and low productivity firms falls to 5.9 percentage points – a ½ percentage point decline (Graph 2). Previous research has shown that this shift is large enough to influence aggregate productivity growth to some extent, particularly if it is sustained over a number of years (e.g. Hambur 2021).
Graph 2
Firm/worker wages are less related to productivity when industry award usage goes up
The above results show that higher use of IA tends to be associated with slower flows of workers to productive firms. But to provide more evidence, I also tested whether high productivity firms appear less willing or able to offer differentiated, higher wages when IA usage is more prevalent. In this case, we would expect to see a weaker relationship between wages and firm-level productivity when IA usage rises.
I ran some simple regressions of wages on firm productivity, allowing the relationship to differ based on the wage-setting mechanisms. I did so by regressing both average wages in the firm and individual workers wages on the firms productivity, while accounting for other firm- or worker-specific factors that can influence wages (e.g. worker age or occupation, firm industry and the strength of the economy).
More precisely, I ran the following the regression:
First, I ran these regressions at a firm level, comparing firms wages (from tax data) to their productivity, and considered whether this relationship differed for firms with differing shares of workers on IA (based on EEH microdata). In this sense, I compared firms that tend to use IA intensively versus those that do not.
Table 2 shows the results. Wages tend to be higher at more productive firms . But the relationship between worker wages and firm productivity is weaker when firms use IA . Based on the coefficients, the relationship between productivity and wages would be one-third smaller for a firm that has all workers on IA, compared with one with all workers on other agreements. This is the case even if firm fixed effects are included, which account for structural firm-specific factors that could drive both wages and the choice of wage-setting mechanism, such as the firms business model.
Base (1) |
Firm fixed effects (2) |
|
---|---|---|
Productivity | 0.091*** | 0.088*** |
(t-stat) | (31.76) | (18.78) |
Productivity × Industry award share | −0.039*** | −0.031*** |
(t-stat) | (−7.5) | (−3.73) |
Controls | ||
Firm FE | N | Y |
Observations | 59,413 | 59,100 |
R-squared | 0.367 | 0.617 |
(a) All regressions include controls for wage-setting mechanism, firm demographics (age and size), and industry and state by year, to account for prevailing economic conditions. *, * and *** show significance at the 10, 5 and 1 per cent level, respectively. Regressions cover 2002–2018. Industry award workers relationship expressed relative to average of individual award or EBA. Source: RBA |
Finally, as a further robustness test, I ran worker-level regressions taking information from the EEH Survey on the workers (ordinary time) wage rate and their wage-setting mechanism. These data provide a one-time snapshot of outcomes for a number of workers in each firm, allowing for comparison of outcomes between firms, or between workers within a firm, but not for a given firm across time.
Table 3 shows the results. Wages tend to be higher at more productive firms . But the relationship between worker wages and firm productivity is again weaker for workers on IA . This finding is robust, even when I allowed the relationship between wages and productivity to differ between occupations (column 2). The evidence was weaker when I included firm fixed effects, and so effectively compared workers on different wage-setting mechanisms within the same firm (columns 3 and 4). However, wage-setting mechanisms tend to be the same for most workers in a given firm, so its not surprising that its hard to find differences within firms.
Base (1) |
Allow occupation specific rent-sharing (2) |
Firm effects (3) |
Firm effects and allow occupation specific rent-sharing (4) |
|
---|---|---|---|---|
Productivity | 0.034*** | – | 0.016** | – |
(t-stat) | (10.60) | (2.25) | ||
Productivity × Industry Award | −0.028*** | −0.018*** | −0.011* | −0.010 |
(t-stat) | (−5.10) | (−3.16) | (−1.94) | (−1.40) |
Controls | ||||
4-digit ANZSCO × productivity | N | Y | N | Y |
Firm FE | N | N | Y | Y |
Observations | 47,586 | 47,586 | 47,325 | 47,325 |
R-squared | 0.670 | 0.678 | 0.786 | 0.789 |
(a) All regressions include controls for worker demographics (quadratic in age, and gender), and wage-setting mechanism, and division, state and 4-digit occupation by year, to account for prevailing economic conditions. *, * and *** show significance at the 10, 5 and 1 per cent level, respectively. Errors clustered at firm level. Regressions cover 2012, 2014, 2018. Industry award workers relationship expressed relative to individual award. EBA interaction not shown. For columns 2 and 4, overall response captured in occupation*productivity controls. Source: RBA |
Overall, the results suggest that where IA use is more prevalent, the relationship between firm-level productivity and wages is weaker. This is unsurprising as these wage rates are set at a national rather than a firm level. This provides further evidence that increased IA use could be associated with less wage differentiation and poaching, and therefore less flow of labour to more productive firms.
Discussion and conclusions
Slower productivity growth has significant negative implications for wages, incomes and living standards. As such, it is crucial to understand why more productive firms have been growing and attracting labour more slowly than in the past. The above results point to one potential factor: the increased use of IA, where all firms offer the same wages no matter how productive they are, is associated with slower flows of labour to productive firms. However, the results do not provide much evidence on why the use of IA has increased, which is crucial in thinking about policy as it is these ultimate causes that will determine whether the trend continues and whether they need to be addressed.
One potential explanation is that some form of frictions have prevented firms from using other mechanisms and offering differentiated wages. For example, the costs and complexities in entering into EBA could have become more significant, relative to the benefits. Alternatively, firms and workers may increasingly be taking IA as a signal of the appropriate wage level. For example, US studies have found that, where there is a benchmark wage for an occupation, firms are more likely to offer similar wages (Cullen, Li and Perez-Truglia 2022).
Another potential explanation is that firms bargaining power may have increased, allowing more of them to lower wages down to the IA level. So it could be that the increases in firms market power have led to increased IA usage and therefore slower reallocation to high productivity firms. In fact, a key argument for minimum wages, such as IA, is that they can mitigate the effects of firms bargaining power, particularly in concentrated markets (Azar et al 2021).
Recent work suggests that firm bargaining power rose as rates of firm creation declined over the 2010s, as this meant less competition for workers and fewer new employment opportunities for workers to leverage in negotiations (Hambur 2023). While there is no evidence linking this to increased use of IA, it does similarly speak to the importance of promoting dynamic and competitive markets where firms compete for workers.
Overall, these results provide further evidence that at least part of the slowdown in productivity growth reflects frictions or other issues that make the economy less dynamic, such that firms have less of an imperative to compete with each other for workers or sales. Increased use of industry minimum wage arrangements is one mechanism through which this has affected economic outcomes, but it is not the only one. Understanding and addressing these issues is important, given slower productivity growth has real implications for wages, incomes and peoples welfare.
Appendix A
I measured productivity as the ratio of value-added to full-time equivalent employees (FTE), where FTE is provided in BLADE based on ABS calculations. Value-added was measured as income less expenses other than labour, depreciation and some other fixed expenses. Value-added was deflated using division-level deflators.
For growth in labour, I measured growth in FTE. Rather than using a standard growth rate, I used the bounded growth rate that is common in the literature:
The advantage of this approach is that it is bounded by −2 and 2, and is an approximation of the log change. While this measure can also accommodate firm entry and exit (2 and −2 respectively), I focused only on existing and continuing firms (the intensive margin of productivity growth).
As noted, for some of the analysis I constructed firm- or division-level industry award wage worker shares. For these shares, I used unweighted counts of workers on industry awards, and on other wage-setting mechanisms. Ideally, I would have used a weighting scheme similar to that used by the ABS in the EEH survey, but weights are not provided in the microdata. Nevertheless, the internally constructed and published division-level metrics appear similar for the overlapping periods and give similar results.
References
Andrews D and D Hansell (2021), Productivity-enhancing Labour Reallocation in Australia, Economic Record, 97(317), pp 157–169.
Azar J, Huet-Vaughn, Emiliano, I Marinescu, B Taska and T von Wachter (2019), Minimum Wage Employment Effects and Labor Market Concentration, NBER Working Paper No 26101.
Bilal A, N Engborn, S Mongey and GL Violante (2022), Firm and Worker Dynamics in a Frictional Labor Market, Econometrica, 90(4), pp 1425–1462.
Card D, AR Cardoso, J Heining and P Kline (2018), Firms and Labor Market Inequality: Evidence and Some Theory, Journal of Labor Economics, 36(S1), pp 13–70.
Cullen ZB, S Li and R Perez-Truglia (2022), Whats My Employee Worth? The Effects of Salary Benchmarking, NBER Working Paper No 30570.
Dustmann C, A Lindner, U Schonberg, M Umkehrer and P vom Berge (2021), Reallocation Effects of the Minimum Wage, The Quarterly Journal of Economics, 137(1), pp 267–328.
Garnero A, F Rycx and I Terraz (2020), Productivity and Wage Effects of Firm-Level Collective Agreements: Evidence from Belgian Linked Panel Data, British Journal of Industrial Relations, 58(4), pp 936–972.
Guertzgen N (2009), Rent-sharing and Collective Bargaining Coverage: Evidence from Linked Employer–Employee Data, The Scandinavian Journal of Economics, 111(2), pp 323–349.
Hambur J (2021), Product Market Competition and its Implications for the Australian Economy, Treasury Working Paper No 2021-03.
Hambur J (2023), Did Labour Market Concentration Lower Wages Growth Pre-COVID?, RBA Research Discussion Paper No 2023-02.
OECD (2019), Negotiating Our Way Up: Collective Bargaining in a Changing World of Work, November.
Productivity Commission (2022), 5-year Productivity Inquiry: A More Productive Labour Market, Interim Report No 6, October.
Sobeck K (2022), Greedy Jobs, Labour Market Institutions, and the Gender Pay Gap, Tax and Transfer Policy Institute Working Paper No 15/2022.
Source: Reserve Bank of Australia [2021]